Antecedents and consequences of college students’ satisfaction with online learning
Main Article Content
We delved into the antecedents and consequences of college students’ satisfaction with online learning. We proposed the antecedents would be interactions, teaching presence, self-management of learning, and academic self-efficacy, and that the consequence would be intention to continue to use online learning. Participants were 236 college students in South Korea who completed an online survey. Our findings suggest that students’ satisfaction with online learning was positively related to the interactions between students and instructor, teaching presence, self-management of learning, and academic self-efficacy. We also found that student satisfaction with online learning positively predicted their intention to continue to use online learning. Thus, our findings in this study provide educators with ways to increase student satisfaction, and add to knowledge about the relationship between students’ satisfaction and their intention to take online courses.
Enrollment in online education courses (e-learning) continues to increase, while, at the same time, enrollment in higher education continues to decline (Seaman et al., 2018). E-learning creates challenges and opportunities for learners, educators, and school administrators (Alshare et al., 2011). In Korea, because of the COVID-19 pandemic, online learning is now positioned as an important format for delivering education. In fact, the worldwide COVID-19 pandemic has catalyzed the emergence of e-learning as a sustainable option for education.
Despite its importance, research on online learning in Korea has been scant. In particular, few researchers have examined the factors that contribute to students’ satisfaction with online learning, or the outcomes of this mode of learning. Moreover, no prior studies of students’ satisfaction with online learning have been conducted in the context of real-time learning (Alshare et al., 2011; Arbaugh & Duray, 2002; Artino, 2007; Bolliger, 2004; Eom & Ashill, 2016; Garrison et al., 2001; Richardson & Price, 2003; Shen et al., 2013). The current research, in contrast, was conducted in the context of learning happening in real time, and we examined the importance of synchronous research in online education. Thus, our study will strengthen the literature by providing insight into online learning environments and student satisfaction.
Literature Review and Development of Hypotheses
Interaction
Moore and Kearsley (1996) suggested that interaction is the most important component of student engagement, be it in traditional face-to-face or in distance learning settings. Interaction in distance learning is deemed to have the following three dimensions (Moore, 1989): First, learner–learner interaction refers to a two-way reciprocal communication between learners. Here, an instructor may or may not be present. In this dimension of interaction, learners can actively exchange their ideas and give feedback to others (T. Anderson, 2003; Moore, 1989). Second, in learner–content interaction the learner elaborates on and reflects upon the subject matter or the content of the course. Thus, this is a one-way process (Moore, 1989). Third, in learner–instructor interaction the learner interacts with the course instructor in two-way communication (Moore & Kearsley, 1996).
In a distance learning environment, among these three dimensions, the learner–instructor interaction is the most important factor for predicting students’ online learning satisfaction (Thurmond, 2003). Battalio (2007) and Bolliger (2004) found that the learner–instructor interaction was the strongest predictor of student satisfaction in both undergraduate and graduates online courses. Thus, in this study, in which our focus was only on the interaction between learner and instructor, we put forward the following hypothesis:
Hypothesis 1: Interactions between students and instructor will be positively related to students’ satisfaction with online learning.
Teaching Presence
Presence refers, in general, to “a person’s sense of being in a place and belonging to a group” (Joo et al., 2011, p. 1656). In looking for predictors of student learning outcomes or performance, researchers have examined teaching presence, social presence, and cognitive presence (T. Anderson et al., 2001; Garrison et al., 2001; Shea et al., 2003). Among these three types of presence, teaching presence in particular has drawn a great deal of attention, especially in the context of online education.
T. Anderson and colleagues (2001) defined teaching presence as teachers designing, facilitating, and providing direction of cognitive and social processes so that their students realize “personally meaningful and educationally worthwhile learning outcomes” (p. 8). Teaching presence has three subdimensions (T. Anderson et al., 2001): First, instructional design and organization refers to preparation of the course content and, thus, includes outlining the online course content, process, and overall structure, and carrying out assessments. It involves the overall design and planning of the online course. Second, facilitating discourse means promoting a learning environment conducive to an active learning process, such as keeping up students’ interest, motivation, and engagement. Third, direct instruction refers to utilizing pedagogical expertise and subject content to enable students to construct their own knowledge.
Concerning the effects of teaching presence in online learning, Caskurlu and colleagues (2020) found a moderately strong positive relationship between teaching presence and learner satisfaction. Furthermore, their results show that in fully online courses, each of the three dimensions of teaching presence predicted student outcomes. Other studies also support there being a strongly positive relationship between teaching presence and student satisfaction (Akyol & Garrison, 2008; Arbaugh, 2008; Khalid, 2014). Hence, we proposed the following hypothesis:
Hypothesis 2: Teaching presence will be positively related to students’ satisfaction with online learning.
Self-Management of Learning
Self-management of learning refers to students’ ability to engage in autonomous learning, and their belief in their own ability to exercise self-discipline (Wang et al., 2009). Researchers often use the term self-management of learning interchangeably with self-directed, independent, or autonomous learning (Regan, 2003). Previous studies have found that self-management of learning has a critical influence on students’ learning outcomes (Abar & Loken, 2010; Chen, 2002; Lounsbury et al., 2009). High levels of self-managed learning lead to higher academic achievement (Chen, 2002) and a better cumulative grade point average (Lounsbury et al., 2009). Researchers have also reported that students who have strong self-management of learning demonstrate an intrinsic motivation to learn; thus, there is a strong relationship between their learning outcomes and satisfaction (Ahmad & Majid, 2010). Hence, a plausible assumption is that students with higher (vs. lower) levels of self-management of learning will be more satisfied with online learning, Therefore, we proposed the following hypothesis:
Hypothesis 3: Self-management of learning will be positively related to students’ satisfaction with online learning.
Academic Self-Efficacy
Self-efficacy denotes an individual’s belief in their capability when engaging in or performing a task (Bandura, 1977). Bandura (1977) suggested that initiation of and persistence in engaging in a task is influenced by self-efficacy. Thus, it is assumed that self-efficacy and persistence have a positive relationship. In an online education context, academic self-efficacy is defined as the confidence of the learners in their learning and performance. Artino (2007), Meece et al. (2006), and Pintrich (1999) all found that self-efficacy was positively related to students’ self-regulation and academic performance. In addition, academic self-efficacy is known to be related to social interaction (Yang et al., 2006).
Researchers have found that students with higher (vs. lower) academic self-efficacy are likely to have more confidence in learning and performance, and are better able to regulate their effort in online learning environments (Shea & Bidjerano, 2010; Yang et al., 2006). Shen and colleagues (2013) identified the dimensions of online learning self-efficacy as Internet self-efficacy, computer self-efficacy, and academic self-efficacy, and found that academic self-efficacy was the most important dimension of the three in explaining students’ satisfaction with online learning. In a similar vein, Artino (2007) suggested that academic self-efficacy was positively related to students’ overall satisfaction with online training. Thus, we posited the following hypothesis:
Hypothesis 4: Academic self-efficacy will be positively related to students’ satisfaction with online learning.
Satisfaction With Online Learning
When it comes to evaluating the goals of both traditional, face-to-face learning courses and online learning courses, researchers should consider cognitive and affective factors. Regardless of which is the learning platform, the course grade is often regarded as an indicator of student achievement. Kuo and colleagues (2014) suggested that a topic worth investigation is student satisfaction, which can be gauged by how students perceive their learning experiences and the value of a course. Prior research has found that student satisfaction was correlated with persistence, course quality, (course) retention, and student success (Allen & Seaman, 2008; Koseke & Koseke, 1991; Moore & Kearsley, 1996; Noel-Levitz Inc., 2011). Extant studies have also found that learners with higher (vs. lower) satisfaction are more likely to have persistence in learning and motivation in pursuing additional online courses (Allen & Seaman, 2008; Biner et al., 1997). Therefore, we proposed the following hypothesis:
Hypothesis 5: Student satisfaction with online learning will be positively related to their intention to continue to use online learning.
Method
Participants and Procedure
Participants were 236 college students from Hongik University, South Korea, who were taking online introductory advertising courses. They completed the survey in return for course credit. Most participants were juniors (38.1%, n = 90), followed by sophomores (37.3%, n = 88), freshmen (16.5%, n = 39), and seniors (8.1%, n = 19). The mean age was 23.2 years (SD = 1.24, range = 20–26 years). Among the students, 33.5% (n = 79) were men and 66.5% (n = 157) were women.
To collect data we created an online survey link through Qualtrics, which provides professional survey software. First, an online survey link (uniform resource locator) was emailed to students enrolled in the class. An informed consent notice was given to students who agreed to participate. Before participants provided their responses, they were directed to provide an outline of what they were learning in the online introductory advertising courses they were taking at the time. The Institutional Research Ethics Board of Hongik University approved this study.
In terms of the order in which students were presented with the scales, they first rated their satisfaction with online learning and their intention to continue to use online learning. Then, they rated interaction, teaching presence, self-management learning, and academic self-efficacy, in that order. Finally, participants answered demographic questions. After completing the online survey, participants were redirected to a webpage containing information on the purpose of this study and a thank-you message.
Measures
In assessing their levels of interaction, participants ranked four statements on a 7-point Likert scale ranging from 1 = strongly disagree to 7 = strongly agree. An example item is “I had frequent positive and constructive interactions with the instructor in this online class.” We adopted this scale from Eom and Ashill (2016), who measured students’ interaction with instructors. Cronbach’s alpha in our study was .84.
Teaching presence was measured with six items rated on a 7-point Likert-type scale ranging from 1 = strongly disagree to 7 = strongly agree. An example item is “The instructor clearly communicated important course goals and course topics.” We adopted this scale from Shea et al. (2003), who measured teaching presence in a face-to-face context, and modified the items for use in the online learning context. Cronbach’s alpha in our study was .89.
We adopted items for self-managed learning from a study by Richardson and Price (2003). Participants’ self-management learning was measured with four items rated on a 7-point Likert-type scale ranging from 1 = strongly disagree to 7 = strongly agree. An example item is “When it comes to learning and studying, I am a self-directed person.” Cronbach’s alpha in this study was .86.
The academic self-efficacy measure comprised five items from Fan and Williams (2010) that are measured on a 7-point Likert scale ranging from 1 = strongly disagree to 7 = strongly agree. An example item is “I’m confident that I can do an excellent job on my tests.” Cronbach’s alpha in this study was .90.
To assess satisfaction with online learning we adapted four items developed by Eom and Ashill (2016) that are measured on a 7-point Likert scale ranging from 1 = strongly disagree to 7 = strongly agree. An example item is “I would recommend this instructor to other students.” Cronbach’s alpha in this study was .80.
Intention to use online learning was measured with three items taken from Malhotra and Galletta (1999) and modified for use in this study. An example item is “I intend to use e-learning to assist my learning.” Ratings are made on a 7-point Likert scale ranging from 1 = strongly disagree to 7 = strongly agree. Cronbach’s alpha in this study was .81.
All measures were translated into Korean. To validate the accuracy of the content we used back-translation to check the scales before the study was conducted. Table 1 shows the reliability values for the various constructs, with average variance extracted values shown on the diagonal. Cronbach’s alpha for the constructs ranged from .81 to .90, indicating acceptable reliability.
Table 1. Discriminant Validity and Correlation Metrics for Measured Variables

Note. N = 236. Values on the diagonal are the square roots of average variance extracted.
Data Analysis
A path analysis was performed with Amos 21.0 to test the structural model.
Results
The correlation results in Table 1 indicate there were significant relationships among the measured variables. As shown in Table 2, the overall indices for the model revealed a poor fit to the data, chi square (χ2) = 25.50, df = 10, p < .001, normed fit index (NFI) = .63, goodness-of-fit index (GFI) = .69, adjusted goodness-of-fit index (AGFI) = .36, comparative fit index (CFI) = .50, incremental fit index (IFI) = .50, relative fit index (RFI) = .24, root mean square error of approximation (RMSEA) = .323.
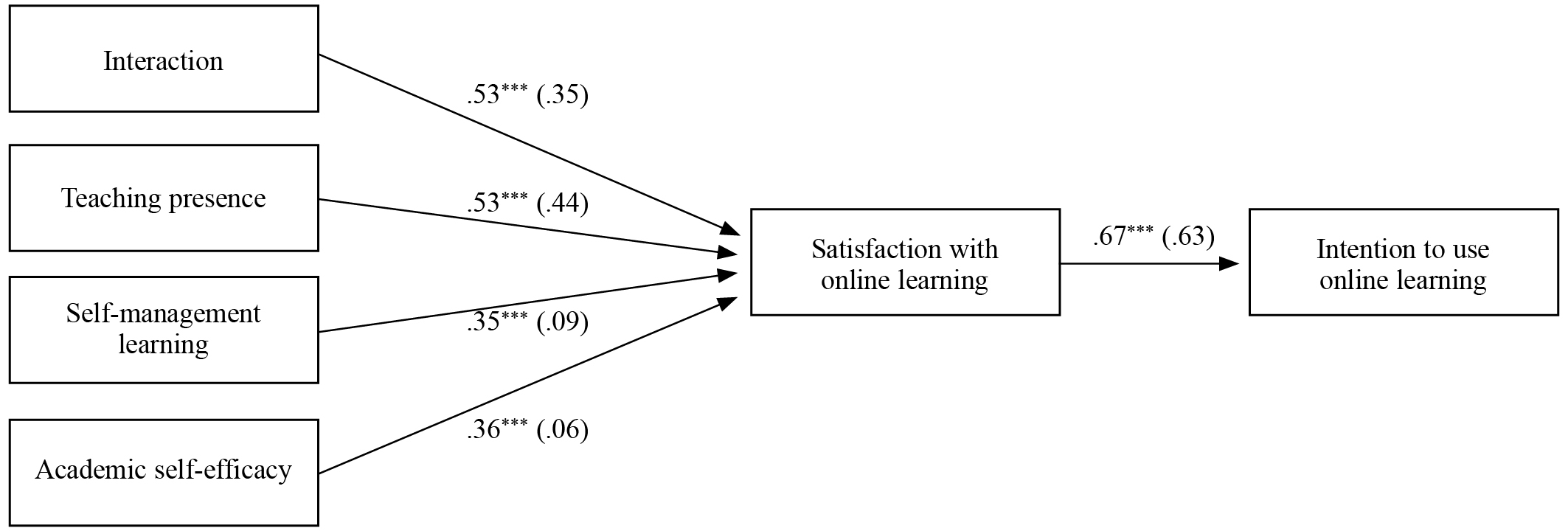
Figure 1. Path Coefficients for the Hypothesized Model
Note. *** p < .001.
Table 2. Original Model

A model is regarded acceptable if NFI and GFI exceed .90, CFI exceeds .93, and RMSEA is less than .08 (Browne & Cudeck, 1992; Byrne, 1994). Thus, the original model was not suitable. As a way of improving the model fit, we examined the modified indices (J. C. Anderson & Gerbing, 1988), which showed that the model fit could be improved by adding covariance paths between the following items or variables: interaction and academic self-efficacy, teaching presence and academic self-efficacy, self-management learning and academic self-efficacy, interaction and teaching presence, teaching presence and self-management learning, and interaction and self-management learning. Therefore, these covariance paths are justifiable. After the model modification, the goodness-of-fit statistics demonstrated that the modified model provided a better fit to the data, χ2 = 33.47, df = 4, p < .001, NFI = .97, GFI = .99, AGFI = .94, CFI = .99, IFI = .99, RFI = .94, RMSEA = .063 (see Figure 2). The parameter estimates for the paths are set out in Table 3.
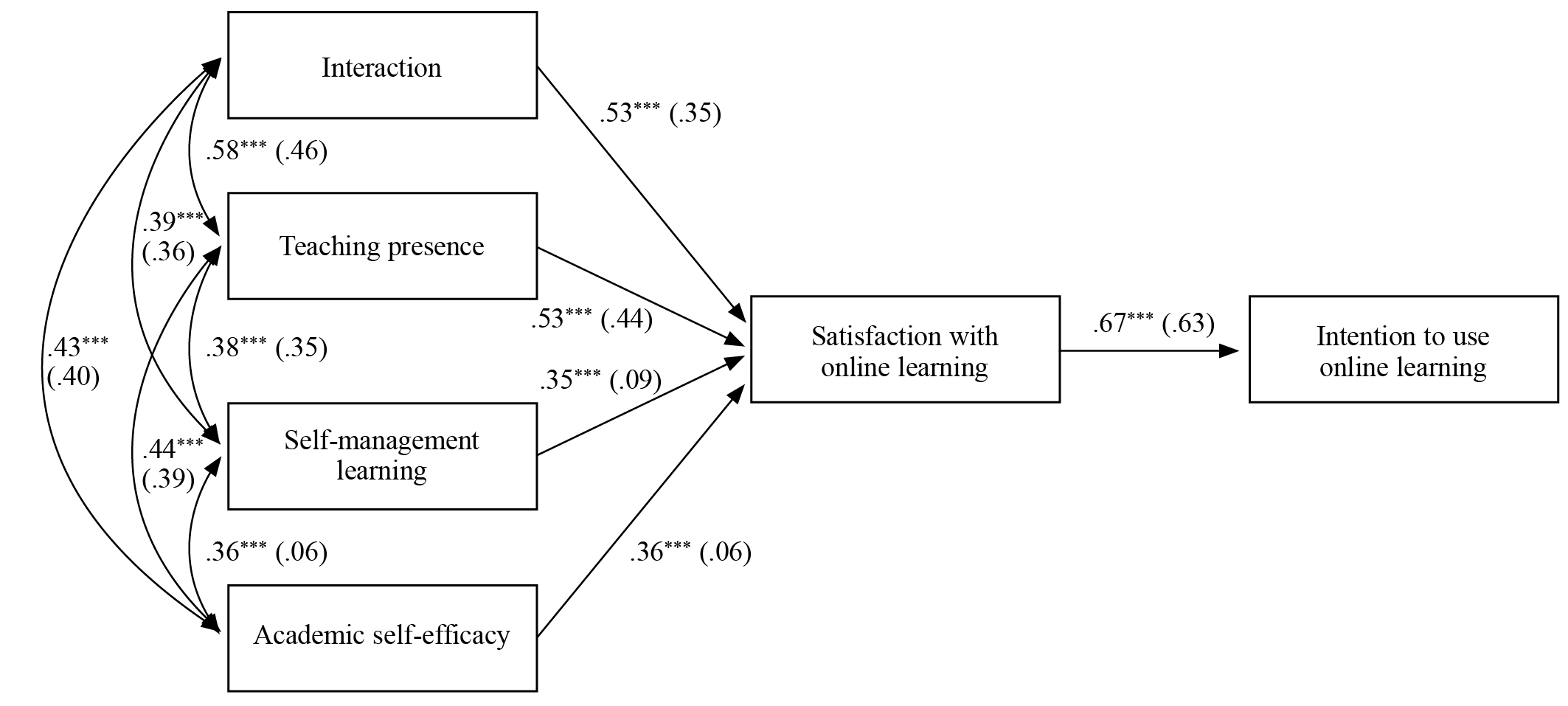
Figure 2. Path Coefficients for the Modified Model
Note. *** p < .001.
Table 3. Modified Model
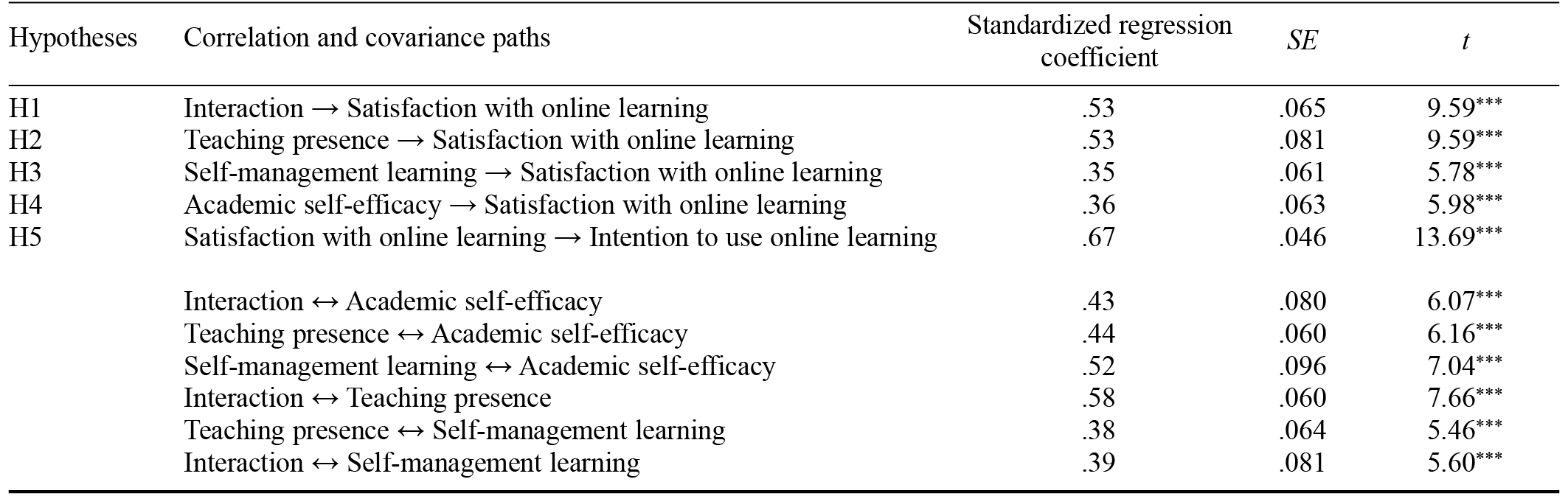
Note. ** p < .001.
According to Anderson and Gerbing (1998), a t value < 2 for each coefficient indicates statistical significance. As shown in Table 2, interaction between students and instructor, teaching presence, self-management of learning, and academic self-efficacy were all positively related to students’ satisfaction with online learning, and students’ satisfaction with online learning was positively related to intention to use online learning. In sum, the study offered support for Hypotheses 1, 2, 3, 4, and 5.
In the modified model we added six additional paths. As shown in Table 3, interaction and academic self-efficacy were positively and significantly related, teaching presence and academic self-efficacy were positively and significantly related, self-management learning and academic self-efficacy were positively and significantly related, interaction and teaching presence efficacy were positively and significantly related, teaching presence and self-management learning were positively and significantly related, and interaction and self-management learning were positively and significantly related.
Discussion
We proposed that interaction with instructors, teaching presence, self-management learning, and academic self-efficacy would antecedents of college students’ satisfaction with online learning, and that a consequence of this satisfaction would be students’ intention to continue to use online learning. Our findings support those reported in prior research. First, we found that student satisfaction with online learning was positively related to interactions with the instructor. There is a great deal of research (see, e.g., Battalio, 2007; Bolliger, 2004; Moore & Kearsley 1996) suggesting that interaction is the most important component of student satisfaction, regardless of the setting (traditional vs. distance) or student level (undergraduate vs. graduate). Thus, to increase satisfaction with online learning, it is important to enhance learners’ perceived interaction with instructors. For instance, while teaching online courses, instructors could enhance this component by providing students with instant feedback, encouraging them to ask questions, and creating small-group discussion opportunities.
Second, our study findings are consistent with prior research suggesting that teaching presence was positively related to student satisfaction with online learning (Akyol & Garrison, 2008; Arbaugh, 2008; Caskurlu et al., 2020; Khalid, 2014). These previous studies found that factors playing a significant role in enhancing students’ satisfaction with online learning include how online courses are designed, how online discourse is encouraged, and how interaction between students is promoted. Thus, online course instructors would do well to incorporate teaching presence into online courses by promoting online discourse and enhancing interaction with students.
Third, we found that self-management of learning was a predictor of students’ satisfaction with online learning. This implies that students’ active engagement is more important in the online learning context than in face-to-face classroom contexts, because students have more control in online classes than in face-to-face classes. While taking online courses, students can choose their own learning pace and have some say in the learning materials employed. Further, these students are able to review what they have learned with learning materials such as study videos. As self-management of learning has a positive impact on students’ learning outcomes (Abar & Loken, 2010; Chen, 2002; Lounsbury et al., 2009) it is important for instructors to remind their online students that they shoulder greater responsibility than their counterparts do in traditional classrooms in completing online courses and achieving desirable learning outcomes.
The fourth finding in our study is that academic self-efficacy was positively related to students’ satisfaction with online learning. Prior research has also found that, of all types of self-efficacy, the most important one in explaining satisfaction with online learning was academic self-efficacy (Artino, 2007; Shen et al., 2013). Several studies have suggested system factors and human factors that could influence learners’ satisfaction with online learning. System factors include system quality and information quality, and human factors include comfort with online learning, self-management of learning, and web self-efficacy (Alshare et al., 2011; Arbaugh & Duray, 2002; Delone & McLean, 1992). Academic self-efficacy could also be deemed a human factor in that it influences how learners perform and succeed in online courses.
Last, our study findings suggest that student satisfaction with online learning positively influenced their intention to continue to use online learning. As student satisfaction with online learning experiences increases, their intention to take more online courses may also increase. It is axiomatic that students’ greater satisfaction with online learning will strengthen their intention to take other online courses.
There are several limitations to this study. First, the sample comprised only college students who are not representative of all online learners in Korea. Thus, the study results are not generalizable to other populations. Online learning takes places not just in university education but also in career schools and hobby courses, among other places. Thus, to broaden the sample representativeness, future researchers should include various age groups. Second, we selected as our research sample students who were currently taking online courses. Thus, the study results are limited to a synchronous online learning environment. Online learning refers to Internet-based courses offered synchronously or asynchronously. We suggest that it would be interesting to compare results from synchronous online courses with those from asynchronous online courses. Third, in terms of variables, we first measured students’ satisfaction with and intention to continue to using online courses (i.e., dependent variables), then measured the variables of interaction, teaching presence, self-management of learning, and academic self-efficacy (i.e., independent variables). The order in which the students were presented with and responded to the measures might have affected our results in this study. Thus, we recommend that in future studies the dependent variables be measured before the independent variables, even in a cross-sectional design.
References
Abar, B., & Loken, E. (2010). Self-regulated learning and self-directed study in a pre-college sample. Learning and Individual Differences, 20(1), 25–29.
https://doi.org/10.1016/j.lindif.2009.09.002
Ahmad, B. E., & Majid, F. A. (2010). Self-directed learning and culture: A study on Malay adult learners. Procedia - Social and Behavioral Sciences, 7, 254–263.
https://doi.org/10.1016/j.sbspro.2010.10.036
Akyol, Z., & Garrison, D. R. (2008). The development of a community of inquiry over time in an online course: Understanding the progression and integration of social, cognitive and teaching presence. Journal of Asynchronous Learning Networks, 12(3–4), 3–22.
Allen, I. E., & Seaman, J. (2008). Staying the course: Online education in the United States, 2008. Sloan Consortium. https://bit.ly/3bmfSs8
Alshare, K. A., Freeze, R. D., Lane, P. L., & Wen, H. J. (2011). The impacts of system and human factors on online learning systems use and learner satisfaction. Decision Sciences Journal of Innovative Education, 9(3), 437–461.
https://doi.org/10.1111/j.1540-4609.2011.00321.x
Anderson, J. C., & Gerbing, D. W. (1988). Structural equation modeling in practice: A review and recommended two-step approach. Psychological Bulletin, 103(3), 411–423.
https://psycnet.apa.org/buy/1989-14190-001
Anderson, T. (2003). Getting the mix right again: An updated and theoretical rationale for interaction. The International Review of Research in Open and Distributed Learning, 4(2).
https://doi.org/10.19173/irrodl.v4i2.149
Anderson, T., Rourke, L., Garrison, D. R., & Archer, W. (2001). Assessing teaching presence in a computer conferencing context. Journal of Asynchronous Learning Networks, 5(2), 1–17.
https://doi.org/10.24059/olj.v5i2.1875
Arbaugh, J. B. (2008). Does the community of inquiry framework predict outcomes in online MBA courses? The International Review of Research in Open and Distributed Learning, 9(2), 1–21.
https://doi.org/10.19173/irrodl.v9i2.490
Arbaugh, J. B., & Duray, R. (2002). Technological and structural characteristics, student learning and satisfaction with web-based courses: An exploratory study of two on-line MBA programs. Management Learning, 33(3), 331–347.
https://doi.org/10.1177/1350507602333003
Artino, A. R., Jr. (2007). Self-regulated learning in online education: A review of the empirical literature. International Journal of Instructional Technology & Distance Learning, 4(6), 3–18. https://bit.ly/3od90CK
Bandura, A. (1977). Self-efficacy: Toward a unifying theory of behavioral change. Psychological Review, 84(2), 191–215.
https://doi.org/10.1037/0033-295X.84.2.191
Battalio, J. (2007). Interaction online: A reevaluation. The Quarterly Review of Distance Education, 8(4), 339–352. https://bit.ly/3howCmo
Biner, P. M., Welsh, K. D., Barone, N. M., Summers, M., & Dean, R. S. (1997). The impact of remote site group size on student satisfaction and relative performance in interactive telecourses. American Journal of Distance Education, 11(1), 23–33.
https://doi.org/10.1080/08923649709526949
Bolliger, D. U. (2004). Key factors for determining student satisfaction in online courses. International Journal on E-learning, 3(1), 61–67. https://bit.ly/3yaeZN3
Browne, M. W., & Cudeck, R. (1992). Alternative ways of assessing model fit. Sociological Methods & Research, 21(2), 230–258.
https://doi.org/10.1177/0049124192021002005
Byrne, B. M. (1994). Structural equation modeling with EQS and EQS/Windows: Basic concepts, applications, and programming. Sage Publications.
Caskurlu, S., Maeda, Y., Richardson, J. C., & Lv, J. (2020). A meta-analysis addressing the relationship between teaching presence and students’ satisfaction and learning. Computers & Education, 157, Article 103966.
https://doi.org/10.1016/j.compedu.2020.103966
Chen, C. C. (2002). Self-regulated learning strategies and regulated learning strategies and achievement in an introduction to information systems course. Information Technology, Learning, and Performance Journal, 20(1), 11–23. https://bit.ly/3yafVB3
DeLone, W. H., & McLean, E. R. (1992). Information systems success: The quest for the dependent variable. Information Systems Research, 3(1), 60–95.
https://doi.org/10.1287/isre.3.1.60
Eom, S. B., & Ashill, N. (2016). The determinants of students’ perceived learning outcomes and satisfaction in university online education: An update. Decision Sciences Journal of Innovative Education, 14(2), 185–215.
https://doi.org/10.1111/dsji.12097
Fan, W., & Williams, C. M. (2010). The effects of parental involvement on students’ academic self-efficacy, engagement and intrinsic motivation. Educational Psychology: An International Journal of Experimental Educational Psychology, 30(1), 53–74.
https://doi.org/10.1080/01443410903353302
Garrison, D. R., Anderson, T., & Archer, W. (2001). Critical thinking, cognitive presence, and computer conferencing in distance education. American Journal of Distance Education, 15(1), 7–23.
https://doi.org/10.1080/08923640109527071
Joo, Y. J., Lim, K. Y., & Kim, E. K. (2011). Online university students’ satisfaction and persistence: Examining perceived level of presence, usefulness and ease of use as predictors in a structural model. Computers & Education, 57(2), 1654–1664.
https://doi.org/10.1016/j.compedu.2011.02.008
Khalid, N. M. (2014). Factors affecting course satisfaction of online Malaysian university students (Published doctoral dissertation). Registry of Research Data Repositories. https://bit.ly/35GFrkn
Koseke, G. F., & Koseke, R. D. (1991). Student “burnout” as a mediator of the stress-outcome relationship. Research in Higher Education, 32(4), 415–431.
https://doi.org/10.1007/BF00992184
Kuo, Y.-C., Walker, A. E., Schroder, K. E. E., & Belland, B. R. (2014). Interaction, Internet self-efficacy, and self-regulated learning as predictors of student satisfaction in online education courses. The Internet and Higher Education, 20, 35–50.
https://doi.org/10.1016/j.iheduc.2013.10.001
Lounsbury, J. W., Levy, J. J., Park, S.-H., Gibson, L. W., & Smith, R. (2009). An investigation of the construct validity of the personality trait of self-directed learning. Learning and Individual Differences, 19(4), 411–418.
https://doi.org/10.1016/j.lindif.2009.03.001
Malhotra, Y., & Galletta, D. F. (1999). Extending the technology acceptance model to account for social influence: Theoretical bases and empirical validation. Proceedings of the 32nd Annual Hawaii International Conference on Systems Sciences (p. 14). Institute of Electrical and Electronic Engineers.
https://doi.org/10.1109/HICSS.1999.772658
Meece, J. L., Anderman, E. M., & Anderman, L. H. (2006). Classroom goal structure, student motivation, and academic achievement. Annual Review of Psychology, 57(1), 505–528.
https://doi.org/10.1146/annurev.psych.56.091103.070258
Meece, J. L., Blumenfeld, P. C., & Hoyle, R. H. (1988). Students’ goal orientations and cognitive engagement in classroom activities. Journal of Educational Psychology, 80(4), 514–523.
https://doi.org/10.1037/0022-0663.80.4.514
Moore, M. G. (1989). Three types of interaction. The American Journal of Distance Education, 3(2), 1–7.
https://doi.org/10.1080/08923648909526659
Moore, M. G., & Kearsley, G. G. (1996). Distance education: A system view. Wadsworth.
Noel-Levitz Inc. (2011). National online learners priorities report. Author. https://bit.ly/3wZwPAF
Pintrich, P. R. (1999). The role of motivation in promoting and sustaining self-regulated learning. International Journal of Educational Research, 31(6), 459-470.
https://doi.org/10.1016/S0883-0355(99)00015-4
Pintrich, P. R. (2004). A conceptual framework for assessing motivation and self-regulated learning in college students. Educational Psychology Review, 16, 385–407.
https://doi.org/10.1007/s10648-004-0006-x
Regan, J. A. (2003). Motivating students towards self-directed learning. Nurse Education Today, 23(8), 593–599.
https://doi.org/10.1016/S0260-6917(03)00099-6
Richardson, J. T. E., & Price, L. (2003). Approaches to studying and perceptions of academic quality in electronically delivered courses. British Journal of Educational Technology, 34(1), 45–56.
https://doi.org/10.1111/1467-8535.00303
Seaman, J. E., Allen, I. E., & Seaman, J. (2018). Grade increase: Tracking distance education in the United States. Babson Survey Research Group. https://bit.ly/3fl8ezj
Shea, P., & Bidjerano, T. (2010). Learning presence: Towards a theory of self-efficacy, self-regulation, and the development of a communities of inquiry in online and blended learning environments. Computers & Education, 55(4), 1721–1731.
https://doi.org/10.1016/j.compedu.2010.07.017
Shea, P., Pickett, A. M., & Pelz, W. E. (2003). A follow-up investigation of “teaching presence” in the SUNY learning network. Journal of Asynchronous Learning Networks, 7(2), 61–80.
https://doi.org/10.24059/olj.v7i2.1856
Shen, D., Cho, M.-H., Tsai, C.-L., & Marra, R. (2013). Unpacking online learning experiences: Online learning self-efficacy and learning satisfaction. The Internet and Higher Education, 19, 10–17.
https://doi.org/10.1016/j.iheduc.2013.04.001
Thurmond, V. A. (2003). Defining interaction and strategies to enhance interactions in web-based courses. Nurse Educator, 28(5), 237–241.
https://doi.org/10.1097/00006223-200309000-00013
Wang, Y.-S., Wu, M.-C., & Wang, H.-Y. (2009). Investigating the determinants and age and gender differences in the acceptance of mobile learning. British Journal of Educational Technology, 40(1), 92–118.
https://doi.org/10.1111/j.1467-8535.2007.00809.x
Yang, C.-C., Tsai, I.-C., Kim, B., Cho, M.-H., & Laffey, J. M. (2006). Exploring the relationships between students’ academic motivation and social ability in online learning environments. The Internet and Higher Education, 9(4), 277–286.
https://doi.org/10.1016/j.iheduc.2006.08.002
Abar, B., & Loken, E. (2010). Self-regulated learning and self-directed study in a pre-college sample. Learning and Individual Differences, 20(1), 25–29.
https://doi.org/10.1016/j.lindif.2009.09.002
Ahmad, B. E., & Majid, F. A. (2010). Self-directed learning and culture: A study on Malay adult learners. Procedia - Social and Behavioral Sciences, 7, 254–263.
https://doi.org/10.1016/j.sbspro.2010.10.036
Akyol, Z., & Garrison, D. R. (2008). The development of a community of inquiry over time in an online course: Understanding the progression and integration of social, cognitive and teaching presence. Journal of Asynchronous Learning Networks, 12(3–4), 3–22.
Allen, I. E., & Seaman, J. (2008). Staying the course: Online education in the United States, 2008. Sloan Consortium. https://bit.ly/3bmfSs8
Alshare, K. A., Freeze, R. D., Lane, P. L., & Wen, H. J. (2011). The impacts of system and human factors on online learning systems use and learner satisfaction. Decision Sciences Journal of Innovative Education, 9(3), 437–461.
https://doi.org/10.1111/j.1540-4609.2011.00321.x
Anderson, J. C., & Gerbing, D. W. (1988). Structural equation modeling in practice: A review and recommended two-step approach. Psychological Bulletin, 103(3), 411–423.
https://psycnet.apa.org/buy/1989-14190-001
Anderson, T. (2003). Getting the mix right again: An updated and theoretical rationale for interaction. The International Review of Research in Open and Distributed Learning, 4(2).
https://doi.org/10.19173/irrodl.v4i2.149
Anderson, T., Rourke, L., Garrison, D. R., & Archer, W. (2001). Assessing teaching presence in a computer conferencing context. Journal of Asynchronous Learning Networks, 5(2), 1–17.
https://doi.org/10.24059/olj.v5i2.1875
Arbaugh, J. B. (2008). Does the community of inquiry framework predict outcomes in online MBA courses? The International Review of Research in Open and Distributed Learning, 9(2), 1–21.
https://doi.org/10.19173/irrodl.v9i2.490
Arbaugh, J. B., & Duray, R. (2002). Technological and structural characteristics, student learning and satisfaction with web-based courses: An exploratory study of two on-line MBA programs. Management Learning, 33(3), 331–347.
https://doi.org/10.1177/1350507602333003
Artino, A. R., Jr. (2007). Self-regulated learning in online education: A review of the empirical literature. International Journal of Instructional Technology & Distance Learning, 4(6), 3–18. https://bit.ly/3od90CK
Bandura, A. (1977). Self-efficacy: Toward a unifying theory of behavioral change. Psychological Review, 84(2), 191–215.
https://doi.org/10.1037/0033-295X.84.2.191
Battalio, J. (2007). Interaction online: A reevaluation. The Quarterly Review of Distance Education, 8(4), 339–352. https://bit.ly/3howCmo
Biner, P. M., Welsh, K. D., Barone, N. M., Summers, M., & Dean, R. S. (1997). The impact of remote site group size on student satisfaction and relative performance in interactive telecourses. American Journal of Distance Education, 11(1), 23–33.
https://doi.org/10.1080/08923649709526949
Bolliger, D. U. (2004). Key factors for determining student satisfaction in online courses. International Journal on E-learning, 3(1), 61–67. https://bit.ly/3yaeZN3
Browne, M. W., & Cudeck, R. (1992). Alternative ways of assessing model fit. Sociological Methods & Research, 21(2), 230–258.
https://doi.org/10.1177/0049124192021002005
Byrne, B. M. (1994). Structural equation modeling with EQS and EQS/Windows: Basic concepts, applications, and programming. Sage Publications.
Caskurlu, S., Maeda, Y., Richardson, J. C., & Lv, J. (2020). A meta-analysis addressing the relationship between teaching presence and students’ satisfaction and learning. Computers & Education, 157, Article 103966.
https://doi.org/10.1016/j.compedu.2020.103966
Chen, C. C. (2002). Self-regulated learning strategies and regulated learning strategies and achievement in an introduction to information systems course. Information Technology, Learning, and Performance Journal, 20(1), 11–23. https://bit.ly/3yafVB3
DeLone, W. H., & McLean, E. R. (1992). Information systems success: The quest for the dependent variable. Information Systems Research, 3(1), 60–95.
https://doi.org/10.1287/isre.3.1.60
Eom, S. B., & Ashill, N. (2016). The determinants of students’ perceived learning outcomes and satisfaction in university online education: An update. Decision Sciences Journal of Innovative Education, 14(2), 185–215.
https://doi.org/10.1111/dsji.12097
Fan, W., & Williams, C. M. (2010). The effects of parental involvement on students’ academic self-efficacy, engagement and intrinsic motivation. Educational Psychology: An International Journal of Experimental Educational Psychology, 30(1), 53–74.
https://doi.org/10.1080/01443410903353302
Garrison, D. R., Anderson, T., & Archer, W. (2001). Critical thinking, cognitive presence, and computer conferencing in distance education. American Journal of Distance Education, 15(1), 7–23.
https://doi.org/10.1080/08923640109527071
Joo, Y. J., Lim, K. Y., & Kim, E. K. (2011). Online university students’ satisfaction and persistence: Examining perceived level of presence, usefulness and ease of use as predictors in a structural model. Computers & Education, 57(2), 1654–1664.
https://doi.org/10.1016/j.compedu.2011.02.008
Khalid, N. M. (2014). Factors affecting course satisfaction of online Malaysian university students (Published doctoral dissertation). Registry of Research Data Repositories. https://bit.ly/35GFrkn
Koseke, G. F., & Koseke, R. D. (1991). Student “burnout” as a mediator of the stress-outcome relationship. Research in Higher Education, 32(4), 415–431.
https://doi.org/10.1007/BF00992184
Kuo, Y.-C., Walker, A. E., Schroder, K. E. E., & Belland, B. R. (2014). Interaction, Internet self-efficacy, and self-regulated learning as predictors of student satisfaction in online education courses. The Internet and Higher Education, 20, 35–50.
https://doi.org/10.1016/j.iheduc.2013.10.001
Lounsbury, J. W., Levy, J. J., Park, S.-H., Gibson, L. W., & Smith, R. (2009). An investigation of the construct validity of the personality trait of self-directed learning. Learning and Individual Differences, 19(4), 411–418.
https://doi.org/10.1016/j.lindif.2009.03.001
Malhotra, Y., & Galletta, D. F. (1999). Extending the technology acceptance model to account for social influence: Theoretical bases and empirical validation. Proceedings of the 32nd Annual Hawaii International Conference on Systems Sciences (p. 14). Institute of Electrical and Electronic Engineers.
https://doi.org/10.1109/HICSS.1999.772658
Meece, J. L., Anderman, E. M., & Anderman, L. H. (2006). Classroom goal structure, student motivation, and academic achievement. Annual Review of Psychology, 57(1), 505–528.
https://doi.org/10.1146/annurev.psych.56.091103.070258
Meece, J. L., Blumenfeld, P. C., & Hoyle, R. H. (1988). Students’ goal orientations and cognitive engagement in classroom activities. Journal of Educational Psychology, 80(4), 514–523.
https://doi.org/10.1037/0022-0663.80.4.514
Moore, M. G. (1989). Three types of interaction. The American Journal of Distance Education, 3(2), 1–7.
https://doi.org/10.1080/08923648909526659
Moore, M. G., & Kearsley, G. G. (1996). Distance education: A system view. Wadsworth.
Noel-Levitz Inc. (2011). National online learners priorities report. Author. https://bit.ly/3wZwPAF
Pintrich, P. R. (1999). The role of motivation in promoting and sustaining self-regulated learning. International Journal of Educational Research, 31(6), 459-470.
https://doi.org/10.1016/S0883-0355(99)00015-4
Pintrich, P. R. (2004). A conceptual framework for assessing motivation and self-regulated learning in college students. Educational Psychology Review, 16, 385–407.
https://doi.org/10.1007/s10648-004-0006-x
Regan, J. A. (2003). Motivating students towards self-directed learning. Nurse Education Today, 23(8), 593–599.
https://doi.org/10.1016/S0260-6917(03)00099-6
Richardson, J. T. E., & Price, L. (2003). Approaches to studying and perceptions of academic quality in electronically delivered courses. British Journal of Educational Technology, 34(1), 45–56.
https://doi.org/10.1111/1467-8535.00303
Seaman, J. E., Allen, I. E., & Seaman, J. (2018). Grade increase: Tracking distance education in the United States. Babson Survey Research Group. https://bit.ly/3fl8ezj
Shea, P., & Bidjerano, T. (2010). Learning presence: Towards a theory of self-efficacy, self-regulation, and the development of a communities of inquiry in online and blended learning environments. Computers & Education, 55(4), 1721–1731.
https://doi.org/10.1016/j.compedu.2010.07.017
Shea, P., Pickett, A. M., & Pelz, W. E. (2003). A follow-up investigation of “teaching presence” in the SUNY learning network. Journal of Asynchronous Learning Networks, 7(2), 61–80.
https://doi.org/10.24059/olj.v7i2.1856
Shen, D., Cho, M.-H., Tsai, C.-L., & Marra, R. (2013). Unpacking online learning experiences: Online learning self-efficacy and learning satisfaction. The Internet and Higher Education, 19, 10–17.
https://doi.org/10.1016/j.iheduc.2013.04.001
Thurmond, V. A. (2003). Defining interaction and strategies to enhance interactions in web-based courses. Nurse Educator, 28(5), 237–241.
https://doi.org/10.1097/00006223-200309000-00013
Wang, Y.-S., Wu, M.-C., & Wang, H.-Y. (2009). Investigating the determinants and age and gender differences in the acceptance of mobile learning. British Journal of Educational Technology, 40(1), 92–118.
https://doi.org/10.1111/j.1467-8535.2007.00809.x
Yang, C.-C., Tsai, I.-C., Kim, B., Cho, M.-H., & Laffey, J. M. (2006). Exploring the relationships between students’ academic motivation and social ability in online learning environments. The Internet and Higher Education, 9(4), 277–286.
https://doi.org/10.1016/j.iheduc.2006.08.002
Table 1. Discriminant Validity and Correlation Metrics for Measured Variables

Note. N = 236. Values on the diagonal are the square roots of average variance extracted.
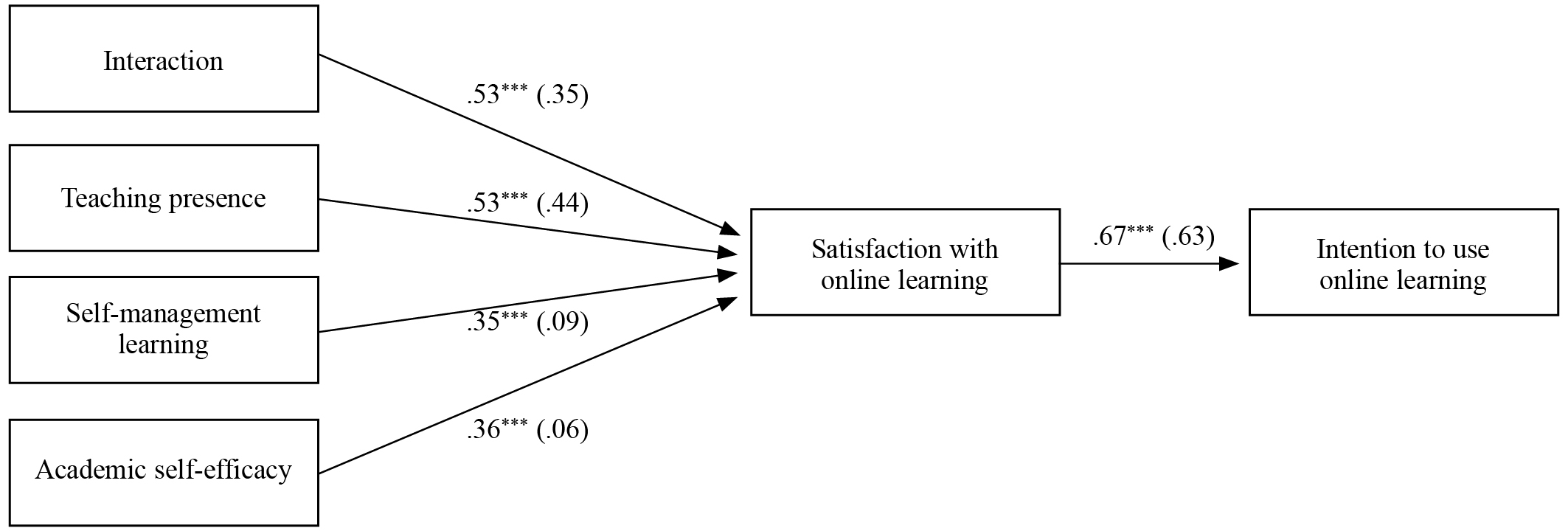
Figure 1. Path Coefficients for the Hypothesized Model
Note. *** p < .001.
Table 2. Original Model

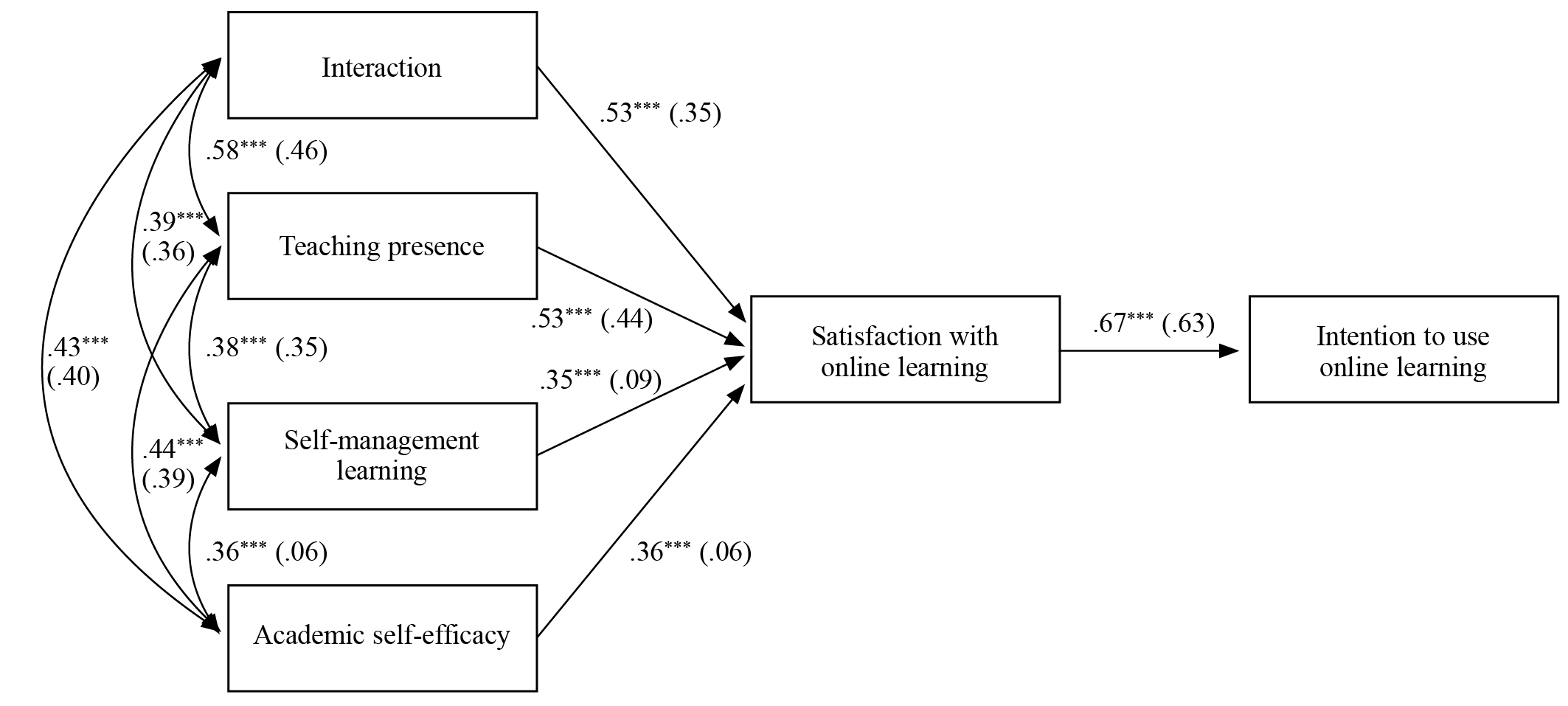
Figure 2. Path Coefficients for the Modified Model
Note. *** p < .001.
Table 3. Modified Model
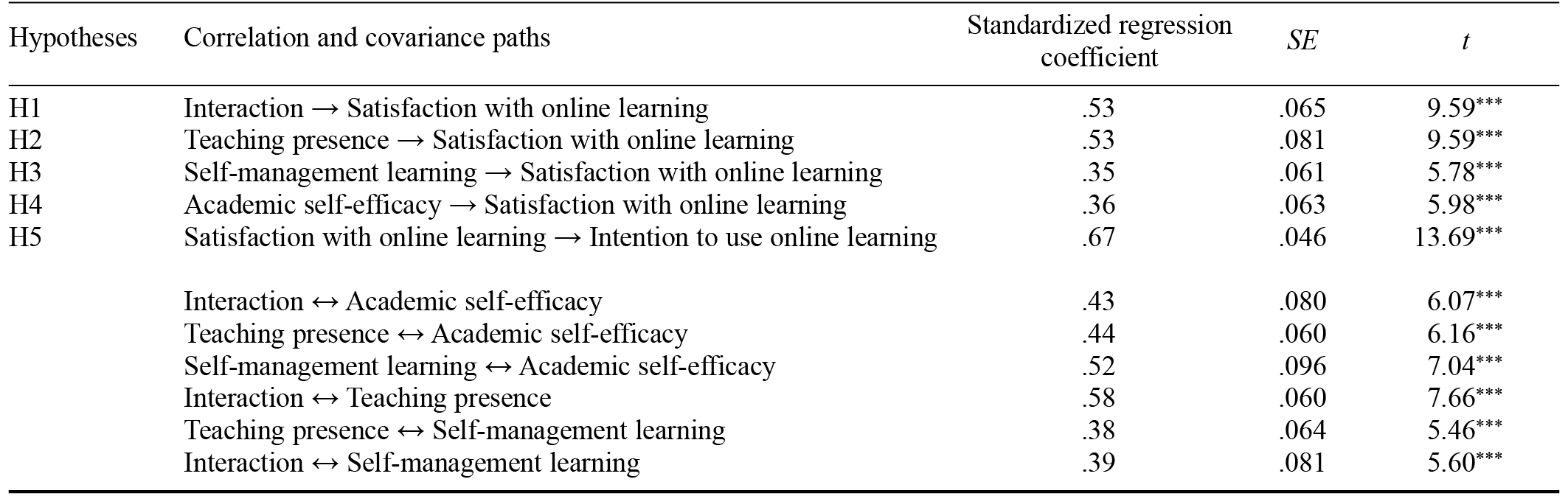
Note. ** p < .001.
Nam-Hyun Urm, School of Advertising and Public Relations, Hongik University, 2639 Sejong-ro, Jochiwon-eup, Sejong, 339-701, Republic of Korea. Email: [email protected]